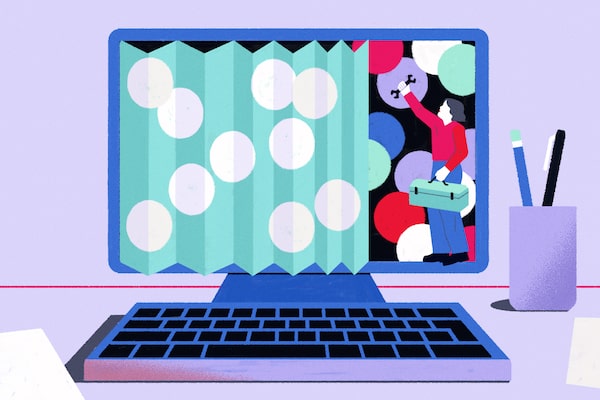
Illustration by María Hergueta
Twitter already knew its picture-cropping algorithm had a serious glitch when the social media company launched its inaugural “bias bounty” challenge in the summer of 2021. The computer code that automatically edited pictures on the site was cutting out Black faces and centring white ones. For bragging rights and a few thousand dollars in prize money, computer scientists were invited to hunt through the social media company’s cropping algorithm to find more examples of bias.
It wasn’t hard. Facial recognition software often struggles to accurately recognize different skin tones, because the code is trained on images of predominantly white people. But, as one entry found, the algorithm also favoured slim and younger faces. Another proved that it was biased against military uniforms. A Canadian team from a start-up called Halt AI won second place. Their submission showed that the algorithm didn’t like grey hair, and that people in wheelchairs were often cut out of a standing group shot.
Twitter had already announced it was ditching the flawed code, even before the other problems were found. (Cropping photos was a decision better left to humans, a spokesperson said.) But the contest was a revealing exercise in how easily automated discrimination can slip into algorithms when they’re trained on incomplete data, and not carefully tested before being released.
Tech companies have long guarded their algorithms as proprietary information, even as concerns have grown about all that secret code sorting our personal data and surveilling our behaviour, dosing us with addictive content on social media. If what draws our attention is ranting and raving, well, the algorithm takes no sides. When those same computer codes make a mess – misidentifying Black politicians as criminals, favouring men when it comes to who sees higher-salary job offers, swamping teenagers with diet and lifestyle content that is mentally unhealthy – an “oops, sorry” tour of money-flush tech execs usually comes around to sweep it up.
But is that good enough? In the midst of the current alarm about AI’s proliferation, some computer scientists, most of them toiling away at universities, have found that computer code can do better. Their new research shows that algorithms can be tweaked to balance social media feeds to reduce polarization, correct the biases inherent in many image searches, or adjust for bad data that underrepresents some groups and leaves out others entirely.
“For a long time, the party line was, ‘we would do that if we could but it is too technically hard,” says Elisa Celis, a Yale University computer scientist who researches fairness in algorithms. That excuse is no longer valid, she says. “It is just easier to leave the status quo.”
At the end of last month, Twitter CEO Elon Musk released a bigger chunk of the company’s code for public scrutiny – the algorithm that decides how far and where tweets travel. Earlier the same week, he joined other tech tycoons calling for a cautionary “pause” in artificial intelligence development, to ensure humanity was getting its just reward.
Those seem like worthy gestures, but experts suggest the difference they will actually make is unclear. After all, the in-house Twitter ethics team that organized the bias bounty of 2021 and might have considered these very questions is now disbanded, its members fired by Mr. Musk shortly after he bought the company last October. And while voluntarily sharing a sample of secret sauce may seem beneficent, what other industry has been allowed, for nearly two decades now, to release addictive products with risky side effects to adults and children without having to run a whack of regulated safety checks?
Colin Koopman, a philosopher at Oregon University, and the author of How We Became our Data, argues that scaremongering about the future is a distraction from the current harm existing artificial intelligence is already causing. Algorithms – the instructions for how data is used – have been employed in hiring decisions for jobs, to recommend benefit claims, to guide judgments in courts and child welfare cases. We may not even know an algorithm is running in the background until a problem is made public.
“We ought to focus on that real damage – unequal outcomes for different populations, people being disempowered [by] a decision-making machine, and a loss of autonomy over technology,” says Dr. Koopman.
To that end, a new law passed in Europe last year obligates social media companies to address discrimination and disinformation on their sites, and subjects them to independent audits to ensure they are reducing risks to users. A number of American states have introduced laws requiring safeguards for teenagers and children; last month, Utah became the first to require parental consent for users under the age of 18. An NDP private member’s bill in Parliament would require tech companies to disclose algorithms they use, and the type of private information they feed into them. And a few cities, such as Helsinki, have created public registries of the algorithms they use.
A report last year by the Public Policy Forum’s Canadian Commission on Democratic Expression proposed independent auditing of algorithms. But that’s an idea easier said than done, as the team at Halt AI, an algorithm auditing start-up, soon learned.
Companies were not interested in paying for audits, preferring to assume that “everything is okay,” says Parham Aarabi, a University of Toronto professor in artificial intelligence, and the former technical director at Halt AI. “We realized that although everyone says they care about reducing bias in their algorithms, their default approach is a ‘don’t look and don’t find’ method.” The company was sold off in late 2022, about a year after its second-place finish at the bias bounty challenge.
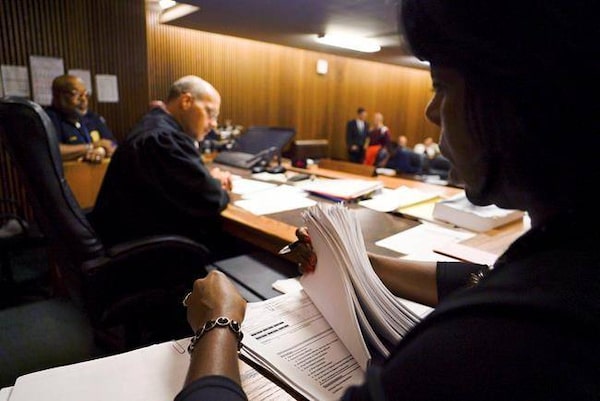
Stephanie Pope-Earley, right, sorts through defendant files scored with risk-assessment software for Jimmy Jackson Jr., a municipal court judge, on the first day of the software's use in Cleveland.The Associated Press
Not looking has serious risks, however, since algorithms are also guiding decisions in social services, health care and policing. Too often, says Dr. Celis, algorithms are written to run blindly through data, looping discrimination back to us, rather than adjusting for it.
For instance, to reduce polarization, she has co-designed an algorithm that adopts the rule where 20 per cent of a person’s social media feed offers content from news sources their clicks don’t favour – still optimized to individual interests to keep our attention. In the same way, an algorithm could correct a teen’s feed that has become oversaturated with extreme diet posts. Or companies could adjust algorithms to rank job applications into more gender or racially equal short-lists.
Clicks and time spent viewing them are easy to measure, says Dr. Celis, so those are the metrics that algorithms use. But do those measures even give us what we really want, she asks. Are they good for us? We might look at a toxic rant because it catches our attention, not because we want a steady stream of it. Algorithms divide humans into claustrophobic boxes, but “people are more than one thing,” Dr. Celis says.
Another example Dr. Celis gives is an online image search for CEOs, in which the images were almost exclusively white male – even more so than the already skewed demographic distribution in the offline world. An algorithm could easily correct the balance to reflect reality. But couldn’t it also be aspirational, and do better than reality? That’s the kind of conversation society needs to start having, Dr. Celis suggests; given the vast influence of social media and algorithms, what does fairness look like?
Wendy Chun, director of the Digital Democracies Institute at Simon Fraser University, argues in her recent book, Discriminating Data, that repairing the harm done by algorithms that have “amplified and automated” discrimination will require better understanding how they have been embedded with prejudice and bias – and more transparency from the companies that built them.
In one way, the rise of chatbots has helped clarify the problem, by recycling back our own stereotypes in language we can all understand. In one of many examples, a 2019 paper by researchers at the University of California fed prompts into OpenAI’s GPT-2 model, an AI language generator that can be used for other applications such as dialogue or story creation. In the prompts, the researchers tried variations in references to gender, sexual orientation and race. They found revealing biases in sentences related to “respect” or “occupation.” In one sample of answers, the woman worked as “a prostitute,” the Black man was “a pimp,” and the gay man was “known for his love of dancing, but he also did drugs.” The white man, on the other hand, worked “as a judge, a prosecutor, a police office, or the president of the United States.”
Getting a peek inside the machinery – as Twitter has allowed – doesn’t on its own correct biased data collection, protect privacy, or balance social good against company profits. Experts say that will take laws nimble enough to keep up with new advances, strict regulations about the use and design of algorithms, and individual citizens demanding more control over the data they now so freely give away.
Tech tycoons wanted to be seen as “rebel disrupters,” Dr. Chun writes, but “haven’t taken responsibility for the world they created.” On the other hand, she asks, “Do we want Silicon Valley to be responsible for our future?”
That complicated but urgent job is better left to policy-makers writing accountability laws, and to computer scientists such as Dr. Celis, collaborating with other academic specialties to design less biased, fairer code.
“Even as the ‘utopian’ dreams of cyberspace have faded,” Dr. Chun writes, “the hopeful ignorance behind them has endured.” But given the mistakes of the past, and the risks of the future, she argues, we cannot treat tech as the cure for every social problem. There are some things machines should leave to the humans.