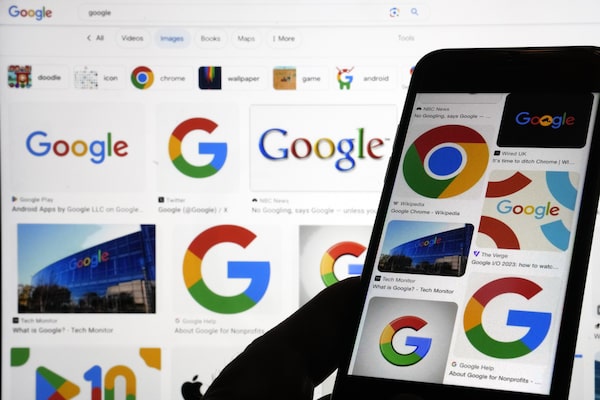
The rollout of Google’s AI-generated search summaries was not without issues as users shared screenshots of bizarre and in some cases potentially harmful results.Richard Drew/The Associated Press
The uneven rollout of Google’s AI-generated search summaries in mid-May was, in a way, entirely predictable. And it’s likely to happen again.
There is a pattern when it comes to the splashy launches of generative artificial intelligence applications from big tech companies. Once these products are in the hands of users, hype quickly gives way to reality, as the apps are found to be error prone and unreliable. A wave of negative media coverage ensues, occasionally followed by an explanation or apology from the offending company.
In Google’s GOOGL-Q case, the company started appending AI Overviews on top of traditional search results, a huge change to its core product. On social media, users quickly started sharing screenshots of the more bizarre results: erroneous information about Barack Obama being the first Muslim president of the United States; nonsensical answers about adding glue to pizza; and potentially harmful advice about eating mushrooms.
Indeed, some companies appear comfortable releasing high-profile but half-baked generative AI products amid intense competitive pressure, even if that means risking embarrassment. The concept of a minimum viable product, wherein a company releases a bare-bones application to test customer needs and demand before developing a full-featured version, is a long-standing one in the tech world. But generative AI companies are pushing that concept to the limit. Depending on whom you ask, the approach is either reckless or a sign that we need to reset our expectations of generative AI.
The release of ChatGPT by OpenAI in November, 2022, touched off an arms race among tech companies. A few months later, Microsoft set the template for janky AI debuts. The tech giant, which has invested heavily in OpenAI, integrated the ChatGPT maker’s technology into its Bing search engine, with lacklustre results. Users widely shared some of the delirious and unhinged responses from the Bing chatbot, which professed love to a New York Times reporter.
Microsoft MSFT-Q quickly made changes, but a fundamental problem with the large language models (LLMs) that underlie chatbots is a propensity for making stuff up. The technology has no capacity for reason or ability to distinguish truth from fiction.
The problems are not limited to text. Earlier this year, Google paused the image generation feature on its Gemini model after users found the system produced historically inaccurate pictures. Prompted to generate illustrations of a German soldier in 1943, for example, the model returned what appeared to be an Asian woman and Black man in Nazi-like uniforms.
Some companies integrating AI into hardware haven’t fared that well, either. Startups Humane and Rabbit released wearable AI-powered devices this year that were meant to supplant smartphones, but both were widely panned in reviews as slow, clunky and severely limited. Even the performance of GPT-4o, which OpenAI debuted in May, is not a big improvement over its predecessor, according to some reviewers.
“They feel like it’s a huge PR advantage to be first. Or not to be last,” said Melanie Mitchell, a computer scientist and professor at the Santa Fe Institute. That may be especially true of Google, which has invested heavily in AI research for years but was seen as slow-moving and cautious after ChatGPT took off. “They’re overreacting in the opposite direction now,” Prof. Mitchell said.
There is another dynamic at play. Sure, it might be embarrassing when the general public plasters social media with examples of misbehaving AI, but it’s also a free form of beta testing. Companies want to better understand how people are using the technology in order to continue to improve it. This is despite the fact that large AI developers have internal safety teams that are supposed to ensure applications will not go off the rails before launch.
Google said as much in a recent blog post explaining what went wrong with its AI-generated search summaries. “We tested the feature extensively before launch,” wrote Google Search head Liz Reid. “But there’s nothing quite like having millions of people using the feature with many novel searches.” The post added that Google has since made more than a dozen technical improvements to its AI overviews.
Ethan Mollick, an associate professor at the Wharton School of the University of Pennsylvania, suggested another factor that is contributing to the rush to release imperfect products. Some AI developers believe the capabilities of the technology are going to progress very rapidly, so the glitches today are seen as “just an interim step,” he said.
Progress has indeed been quite rapid. Just a few years ago, LLMs were far more likely to ramble and spout nonsensical answers than today’s models. Seen through that lens, it’s understandable why developers might not stress too much about an AI summary advising you to eat rocks for the nutritional benefits. It will soon be yesterday’s problem, the thinking goes.
That doesn’t mean progress will continue at the same rate or that the reliability issues with AI are easily fixable. Issues around cost, computing capacity and the availability of data to train new large AI models could constrain development, and there are already signs that the pace of progress is slowing down. A report released earlier this year by Stanford University noted that progress on various benchmarks used to assess the proficiency of AI has “stagnated in recent years, indicating either a plateau in AI capabilities or a shift among researchers toward more complex research challenges.”
Expecting perfection from AI, however, is the wrong approach, according to Dr. Mollick. (It’s also not a standard we apply to colleagues or ourselves.) “AI, even with errors, often beats humans,” he said. On his Substack, Dr. Mollick has suggested a more appropriate measurement is what he dubs the Best Available Human. “Would the best available AI in a particular moment, in a particular place, do a better job solving a problem than the best available human that is actually able to help in a particular situation?” he wrote. There are situations in which that might be the case.
Last fall, Dr. Mollick and his colleagues worked on a study with the Boston Consulting Group to gauge how OpenAI’s GPT-4 could help – or hinder – consultants with various tasks. Overall, people equipped with AI were significantly more productive and produced higher-quality results when it came to creative tasks, such as proposing ideas for a new type of shoe, and writing and marketing tasks, such as drafting news releases.
But on a task that was designed to be beyond the capabilities of AI (a business analysis involving spreadsheet data and interview notes), consultants who relied on GPT-4 performed worse and had less accurate answers. “Outside the frontier, AI output is inaccurate, less useful and degrades human performance,” according to the study.
One take-away is that people can be led astray by generative AI without a thorough understanding of its capabilities and limitations, which is why the rush to release new applications is concerning for some experts.
Prof. Mitchell said there could be a paradoxical outcome from how we use applications such as ChatGPT. If the error rate is in the ballpark of 50 per cent, we will be less likely to trust these systems and double-check the output. If the error rate is more like 5 per cent, we might not even think twice, allowing inaccuracies to slip through. “That better system is in some ways more dangerous than the worst system,” she said.
Until the accuracy and reliability issues are fundamentally fixed – a big if – we can expect to see many more rocky AI debuts in the future.