
Supercomputers stuffed with GPUs in sprawling data centres, like Narval, a supercomputer at Calcul Québec, are crucial to building an AI model.Stéphane Brügger/Supplied
The 212th most-powerful computing system in the world is located in a plaza in Vaughan, Ont., next to an A&W restaurant. There is no signage indicating that inside are hulking machines whirring away on astrophysics calculations or running algorithms for artificial intelligence, only a piece of paper affixed to the front door that reads “Unit 7.”
Down a white brick hallway inside is a computing cluster called Niagara, which is tailored to run large jobs for academics across the country in an array of fields, from climate research to chemistry to biology. The Vector Institute, the AI hub in Toronto, locates most of its 1,000 graphics processing units (GPUs) here too, the coveted chips commonly used in AI. The room is lined with tall black obelisks containing central processing units (CPUs), GPUs and ungainly knots of cables. The incessant whirring of cooling fans is nearly deafening, like being inside a very large vacuum cleaner, while the heat radiating off the back of a single server rack is like the blast from a hot oven.
There are five such computing host sites across the country (others are in British Columbia and Quebec) built to service university and college researchers. The country’s other two AI institutes, Mila in Quebec and Amii in Alberta, have their own limited resources, but rely heavily on this national computing infrastructure, too. The problem is that none of this rapidly aging computing equipment is enough to meet demand, and it amounts to a fraction of the processing power that exists elsewhere in the world. Before falling to No. 212 on a list of the top 500 most capable computing systems in the world, Niagara was ranked No. 53 when it launched in 2018.
Yoshua Bengio, founder and scientific director of Mila Quebec AI Institute, in May 2023. Though it has its own limited resources, Mila also relies heavily on national computing infrastructure.Christinne Muschi/The Canadian Press
In the AI field, the computing needs are particularly acute. The physical infrastructure powering AI – what people in the industry call “compute” – is often invisible to the rest of us. AI is in the cloud, right? But the rows and rows of supercomputers stuffed with GPUs in sprawling data centres are crucial. Training or building an AI model, especially the large language models (LLMs) that underlie chatbots such as ChatGPT, requires immense computing power. Running an AI application, such as asking ChatGPT a question, has different requirements, but these needs escalate with use.
There is huge global demand for computing equipment, especially GPUs, and researchers and entrepreneurs want access to powerful systems to make scientific discoveries and build businesses. That’s becoming harder to do in Canada.
The country has championed itself as a bastion for AI students and researchers, and the federal government prioritized attracting talent when it launched the Pan-Canadian Artificial Intelligence Strategy in 2017. The initial $125-million in funding supported three AI research hubs, each paired with a luminary in the field – Geoffrey Hinton, Yoshua Bengio and Richard Sutton. But a significant underinvestment in compute is now threatening Canada’s AI talent advantage.
“We still have one of the greatest talent concentrations in AI in the world. But we are in danger of losing it because now there’s so much money being invested in these large systems, mostly in the U.S., that the brain drain is probably coming back,” said Prof. Bengio, the scientific director at the Mila AI institute and professor at the Université de Montréal. “The tendency of local graduates to go to the U.S. for jobs, I can see that in my group.”
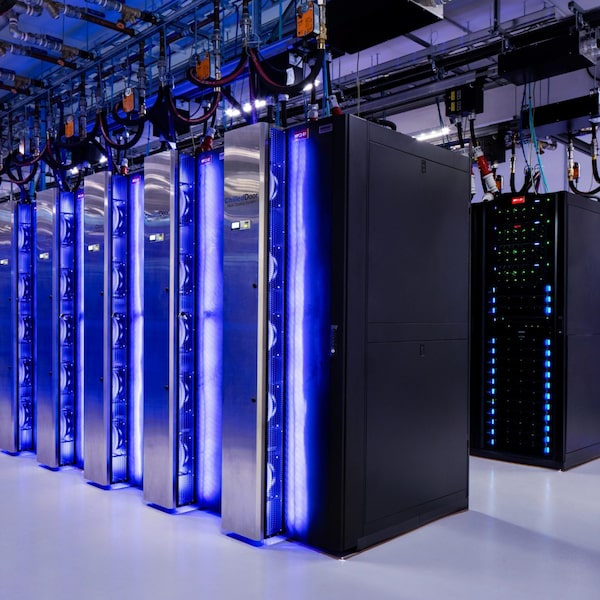
When Calcul Québec’s Narval came online in 2021, it was ranked the 83rd most powerful supercomputer system in the world. In just two years, it dropped to No. 134.Stéphane Brügger/Handout cleared
Canada is already slipping. The country is ranked fifth in the world for its AI capacity, according to the Tortoise Global AI Index published last year, which measures countries based on a variety of factors. But when ranked on infrastructure, Canada fell from 15th to 23rd place compared with the previous study. “It should be a wake-up call,” said Tony Gaffney, chief executive at the Vector Institute. One of the first questions researchers ask him these days is: “What compute can you provide me with?”
The past year has seen a flurry of activity in other countries. The United States, Japan, Germany and Britain are pouring money into shoring up domestic semiconductor supply chains, building new supercomputers and helping companies access compute capabilities. Since March, 2023, Britain has announced about £1.7-billion ($2.9-billion) to expand compute access and build infrastructure. By comparison, Canada announced $40-million to boost AI compute in 2021.
People from across the AI ecosystem are calling on Ottawa to spend big – at least $1-billion to start, and possibly up to $10-billion over a number of years – to catch up, and they hope the coming federal budget will contain such measures. Important questions such as what specifically to build, how much capacity Canada needs and what a long-term sustainable funding model looks like are still unanswered, however. The message is simple: Start building, fast.
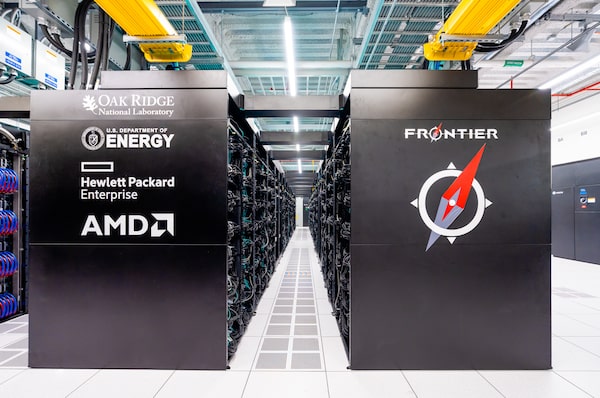
The Exascale-class HPE Cray EX Supercomputer at Oak Ridge National Laboratory in Tennessee.OLCF at ORNL/Handout cleared
Before we proceed, we have to talk about FLOPS. In the simplest terms, a floating point operation (or FLOP) is a mathematical operation performed by a computer. The best smartphones on the market can manage a trillion floating-point operations per second, whereas the most powerful computer in the world, the Frontier system located at the Oak Ridge National Laboratory in Tennessee, can manage a million trillion FLOPS. That’s a one followed by 18 zeros, a number that’s also known as an exaFLOP. Systems capable of such power are referred to as exascale computers, and they are very expensive and very rare. The first European exascale system, which is called Jupiter and located in Germany, is scheduled to fire up later this year.
So where does Canada stand in the FLOP measuring contest? Nowhere, really. Excluding private and internal government systems, the most powerful computing cluster here is called Narval, which is located in Quebec and available to academics. When Narval came online in 2021, it was ranked 83rd in the world. In just two years, it dropped to No. 134. The 10 most powerful systems in the world have achieved 16 to 200 times Narval’s processing power. (Private companies often don’t always disclose their computing resources for competitive reasons.)
More computing power is necessary these days because generative AI models have grown astronomically bigger. LLMs are built on huge volumes of data and require lots of dedicated processing power for training, the process of sifting through that massive ocean of data to find patterns and ultimately make predictions. Between 2015 and 2022, the compute used to train large-scale AI models doubled every 10 months or so, according to a study by Epoch AI. Microsoft Corp. spent several hundred million dollars to build a supercomputer exclusively for San Francisco’s OpenAI after first investing in the company in 2019. When the system came online, it ranked among the top five most powerful in the world.
Compute was not much of a consideration when Canada unveiled its national AI strategy in 2017. “It was still debatable how much you needed,” said Mila CEO Valerie Pisano, who called compute the “weak link” in Canada’s strategy. Researchers have been discussing the need for more capacity for years, though. “We’ve heard it a lot, that the investment in compute to really drive forward Canadian AI leadership still needed to happen,” said Cam Linke, CEO of Amii in Edmonton.
The handful of computing clusters in Canada for academia are overseen by a non-profit organization called the Digital Research Alliance of Canada (DRAC), which is funded by the federal government. A 2021 DRAC report noted that computing needs were growing “phenomenally” and that GPU demand was five times oversubscribed. Canada trailed all of its G7 peers in terms of aggregate computing power, according to the report, and ranked second last when adjusted for gross domestic product. DRAC recommended that capacity should at least be doubled – and that was before the era of generative AI.
Funds have been allotted – for years – to pay for upgrades, but the process has been absolutely glacial, and procurement has only recently got under way. The federal government set aside $375-million in the 2018 budget to establish DRAC and upgrade computing systems. In the 2021 budget, the government announced $40-million over five years to support compute for the three AI institutes.
Even so, the funding earmarked years ago is not nearly sufficient. “The investments we’re making to the existing core sites are really to shore up aging infrastructure right now to make sure that it’s functional,” said George Ross, DRAC’s chief executive. After upgrades, the total processing power will be only about 10 per cent of a single exascale computer, he said.
Ottawa urged to move quickly on AI law to safeguard technology
The Vector Institute started experiencing capacity constraints recently alongside the growth in generative AI. “This is a new phenomenon. It’s really in the last couple of years that the demand is far outstripping supply,” said chief information officer Ben Davies. Some of the 700 researchers serviced by Vector’s cluster want to pursue work on very large AI models, but the institute simply doesn’t have enough compute available. Just training one LLM, for example, could require tens of thousands of GPUs, which is more than 10 times as many as Vector has today.
The Niagara cluster, which supports research across academic fields, has the same problem. “The truth is we’re always overcapacity,” said Daniel Gruner, the chief technology officer at the SciNet High Performance Computing Consortium, which oversees the cluster. Every year, there is an application process in which researchers submit estimates of what they need to complete their work. “A lot of people know that we don’t have enough resources so they don’t even bother applying,” Mr. Gruner said.
Others will scale down their research because of a lack of resources, partner with researchers in another country or try to get access through a commercial cloud provider such as Microsoft, Google or Amazon.com Inc. As a result, scientific possibilities can be foreclosed. “The idea is to enable people to use their ingenuity so they can do things that are far out and different,” Mr. Gruner said. “If you don’t support basic research, you don’t get anywhere.”
The private sector has struggled with compute access, too. David Katz, a partner at Radical Ventures in Toronto, spends part of his time helping portfolio firms connect with cloud service providers. As recently as 2021, that meant negotiating price. But within a year, he was negotiating access, so great was demand.
There was another complicating factor, too. The big cloud companies, such as Microsoft, Google and Amazon, are also developing AI internally, meaning they have to balance their own needs with those of their customers. Meanwhile, Mr. Katz could see compute costs for some companies were rapidly escalating. “That really opened my eyes that this is not even a choice of what chips to give to who at the customer level,” he said. “It’s the whole ecosystem. Everyone is vying for these chips.”
Aidan Gomez, co-founder and CEO of Toronto-based Cohere, speaks during a conference in Toronto in February. Cohere is the country’s only player in building foundational large language models (LLMs).Arlyn McAdorey/The Canadian Press
Toronto-based Cohere, which is backed by Radical Ventures, is the country’s only player in building foundational LLMs. Founded in 2019, the company was able to develop its models thanks to an agreement with Google to use its tensor processor units, custom circuits made by Google specifically for machine learning. Compared with its larger competitors, Cohere appears to be at a disadvantage when it comes to computing infrastructure. OpenAI has billions of dollars from Microsoft and its own supercomputer, while Google and Meta Platforms Inc. already earn billions from massively profitable advertising businesses, money that can be ploughed into more compute. (Cohere declined an interview for this article.)
“This is the first time in my experience where just having money isn’t a solution to the problem,” said Joshua Pantony, CEO of Boosted.ai in Toronto, which makes generative AI tools for asset managers. Compute is the company’s biggest expense, and Mr. Pantony has had to rely on established relationships with cloud computing firms to get access. “If you go to a cloud provider you haven’t been working with for a long time, you get put on a wait list and you often have to precommit the amount of compute that you want to spend, which is challenging,” he said.
His company is in a better position than others, since it has raised a total of US$46-million in funding, but access to compute remains a limiting factor. “There are advancements we could make from an R&D standpoint where we’re not building as quickly as I’d like to,” he said.
Compute is an even bigger challenge for younger companies that haven’t raised as much venture capital, and some people in the field are concerned about the implications. “There is a risk of startup companies deciding they’re going to move to a different jurisdiction to get affordable access to these computing technologies,” said Elissa Strome, executive director of the Pan-Canadian AI Strategy at the Canadian Institute for Advanced Research.

Dave King co-founded Calgary-based Denvr Dataworks, which builds and manages infrastructure and software for clients to run AI workloads.Denvr Dataworks/Supplied
Some entrepreneurs saw the compute crunch coming early. Dave King co-founded Denvr Dataworks in 2018, though the company did not launch its products until January, 2023. Denvr, based in Calgary, builds and manages infrastructure and software for clients to run AI workloads. “If you looked at the trajectory of what was coming, we said something’s gotta give,” Mr. King said.
Business has been booming. Among Denvr’s customers is Stability AI, a big player in generative AI that makes tools to produce images, audio, text and video. Canada accounts for only a small portion of Denvr’s business today. “Canadians are behind, and most of our demand came from the United States in our first full year,” Mr. King said. That’s true even of companies that should have the means to spend on GPUs, such as financial institutions. “We find that their clusters for artificial intelligence are very tiny,” he said. Here, a large firm might have five machines whereas a U.S. financial institution could have as many as 1,000.
With public sector researchers and private companies both facing compute challenges, and with other countries investing to address their own issues, the solution for Canada is obvious to Mr. King: “We need to build supercomputers.”

Nvidia founder and CEO Jensen Huang speaks during the company's annual AI conference in March 2024. Industry Minister François-Philippe Champagne signed a letter of intent with Nvidia to “explore opportunities to work together on creating AI computing power in Canada.JOSH EDELSON/Getty Images
The good news is that if Canada wants to address its dearth of compute, it can simply copy and adapt what other countries have done. There are a couple of immediate steps the federal government can take.
Graham Dobbs, a senior economist at the Dais think tank at Toronto Metropolitan University, said Ottawa could purchase compute access on commercial cloud providers, and develop a process to parcel it out to industry. “It’s a patch fix,” he said, “because we’re still paying hyperscalers and we’re locked into that ecosystem.” The government could also commit to buying GPUs directly from manufacturers, so that the country is guaranteed a certain amount of chips each time there is a new release.
The federal government has made some preliminary, if vague, gestures in that direction. In January, Canada and the United Kingdom announced a memorandum of understanding about “exploring opportunities” to secure compute access for AI researchers and companies. Industry Minister François-Philippe Champagne then signed a letter of intent with Nvidia Corp., whose GPUs are in high demand, to “explore opportunities to work together on creating AI computing power in Canada.”
A spokesperson for Mr. Champagne declined to provide further details. “Innovation, Science and Economic Development Canada recognizes the evolving needs of researchers and industry in Canada as it relates to compute capacity for AI, and the need to keep pace with the rapid developments,” said spokesperson Audrey Champoux in an e-mail.
Many people The Globe and Mail interviewed have spoken to government about the need for compute, and said that officials understand the importance of the issue. But how Ottawa will respond is unclear. A longer-term solution, beyond the government purchasing compute access, would be to build up domestic supercomputing capacity, ensuring that academic researchers and companies can train and run AI models.
Many countries have recognized the importance of AI infrastructure to not only support research and commercialization, but as a sovereignty play, reducing dependence on foreign companies and politically fraught supply chains. The U.S. is spending US$52-billion to bolster semiconductor manufacturing, while Japan has allocated US$13-billion for its chips sector. Britain’s plan involves building three supercomputers – including an exascale system by 2025 – which would increase capacity by 30 times. “We just don’t want to be in a position where we’ve got to feed on the scraps of other competitors and countries,” said Mr. Gaffney at the Vector Institute.
A worker moves a wafer bank at the NXP semiconductors computer chip fabrication plant in Nijmegen, Netherlands. The past year has seen a flurry of activity in other countries, which are investing in domestic semiconductor supply chains.Piroschka Van De Wouw/Reuters
Putting a broad program of measures in place, including buying access in the short term and building up infrastructure in the long term, is where costs start to climb. Over several years, a national strategy could be as much as $10-billion. The government doesn’t have to foot the entire bill, said Ms. Pisano at Mila, adding that Canada could work with other countries to build and share compute resources. “It’s not realistic to think we’re all going to put $10-billion on the table,” Ms. Pisano said. “The question I’d be posing is: Do we do it alone? Or do we do it with others?”
Of course, when the government seizes on an issue, it risks overdoing it. This is the same federal Liberal government that is hell-bent on turning Canada into an electric-vehicle superpower, doling out billions of dollars in subsidies to foreign companies to develop an industry with prospects that are not entirely certain. With AI, the generative variety in particular is driving demand for compute, as not all applications are as resource-intensive.
And for all of the hype and excitement, Canadian companies have been slow to adopt generative AI, which is still a nascent, unreliable and expensive technology that has to prove its worth. There are environmental considerations, too, as data centres and supercomputers consume large amounts of energy and water. Plus, the strong demand for compute should be incentive enough for cloud companies to build out more infrastructure to service the private sector, including in Canada. Supporting academic research is one thing. But do we really need to spend more money to support for-profit companies developing AI?
There are some potential economic benefits to consider. In 2020, Hyperion Research found that every dollar invested in high-performance computing generated $507 in revenue and $47 of profit or cost savings. With generative AI specifically, the Conference Board of Canada estimated in February that the technology could add close to 2 per cent to Canada’s gross domestic product, assuming businesses adopt it. That’s not nothing, but it’s also not “life-changing,” according to Mr. Dobbs. “It’s really a judgment call on whether we try to keep up,” he said of building more compute.
Mr. Pantony at Boosted.ai said the government doesn’t necessarily have to intervene, but it’s difficult to stay on the sidelines when other countries are making moves. “You may run into a situation where it is no longer the invisible hand of the market controlling things,” he said.
There are ways for Canada to address its computational shortcomings without spending billions up front. Ryan Grant, an assistant professor at Queen’s University, has spent his career helping build some of the largest supercomputers in the world. He left the country to work in the U.S. in 2012 and served as a principal technical staff member at a national research lab, but returned home in 2021 to develop supercomputing talent here. “I knew that AI was going to be a major stumbling block in the future,” he said. “People who had experience working on the world’s largest systems didn’t seem to exist in Canada.”
Prof. Grant advocates for building a supercomputer tailored for training AI models – that’s where the need is greatest – as opposed to running them. The government would kick in the funds to get it built, and companies would pay to access it below commercial rates. That money can be invested back into maintaining the system and paying for upgrades. “AI is moving so quickly right now that you need to refresh every two or three years,” he said. Researchers in the public sector could make use of excess capacity for free, or have capacity set aside at a reduced rate.
When it comes time to run AI applications commercially, companies would still have to buy access through a cloud provider. Prof. Grant said that might be a challenge, but it’s nevertheless easier than finding dedicated resources to train models.
Canada could spend between $250-million and $350-million on such a supercomputer. Building one giant system (or more) of Herculean strength comes with problems beyond the price tag. The most advanced systems in the world require more staff to operate them, use equipment that hasn’t necessarily been proven in the field and come with more processing power than a country of our size knows what to do with. Canada should aim to have a system that can rank in the top 30 supercomputers in the world, not the top five. “The goal is to be state-of-the-art so you’re on the cutting edge, but not the bleeding edge,” Prof. Grant said.
In many ways, Canada has already done the hard part when it comes to building an AI ecosystem: attracting talent in the first place. Building the infrastructure to support those people should be comparatively easy. “It’s like we figured out how to build the best car in the world,” Prof. Grant said, “but now we need the factory and the production line to make that into real dollars.”